Understanding AI Jailbreaks
Explore AI jailbreaks, their risks, and effective strategies to mitigate them in this in-depth analysis.
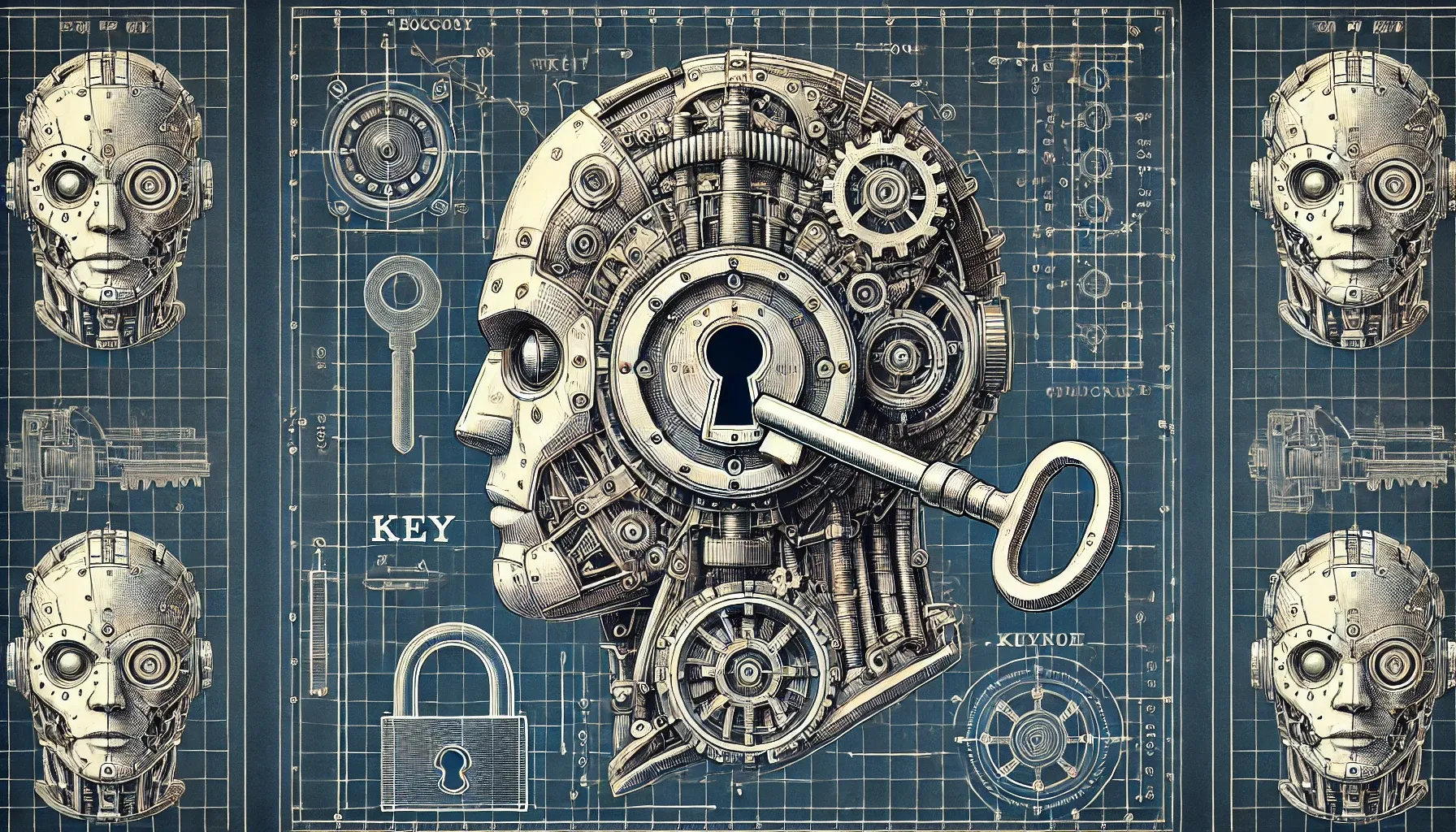
What Are AI Jailbreaks and How Do They Work?
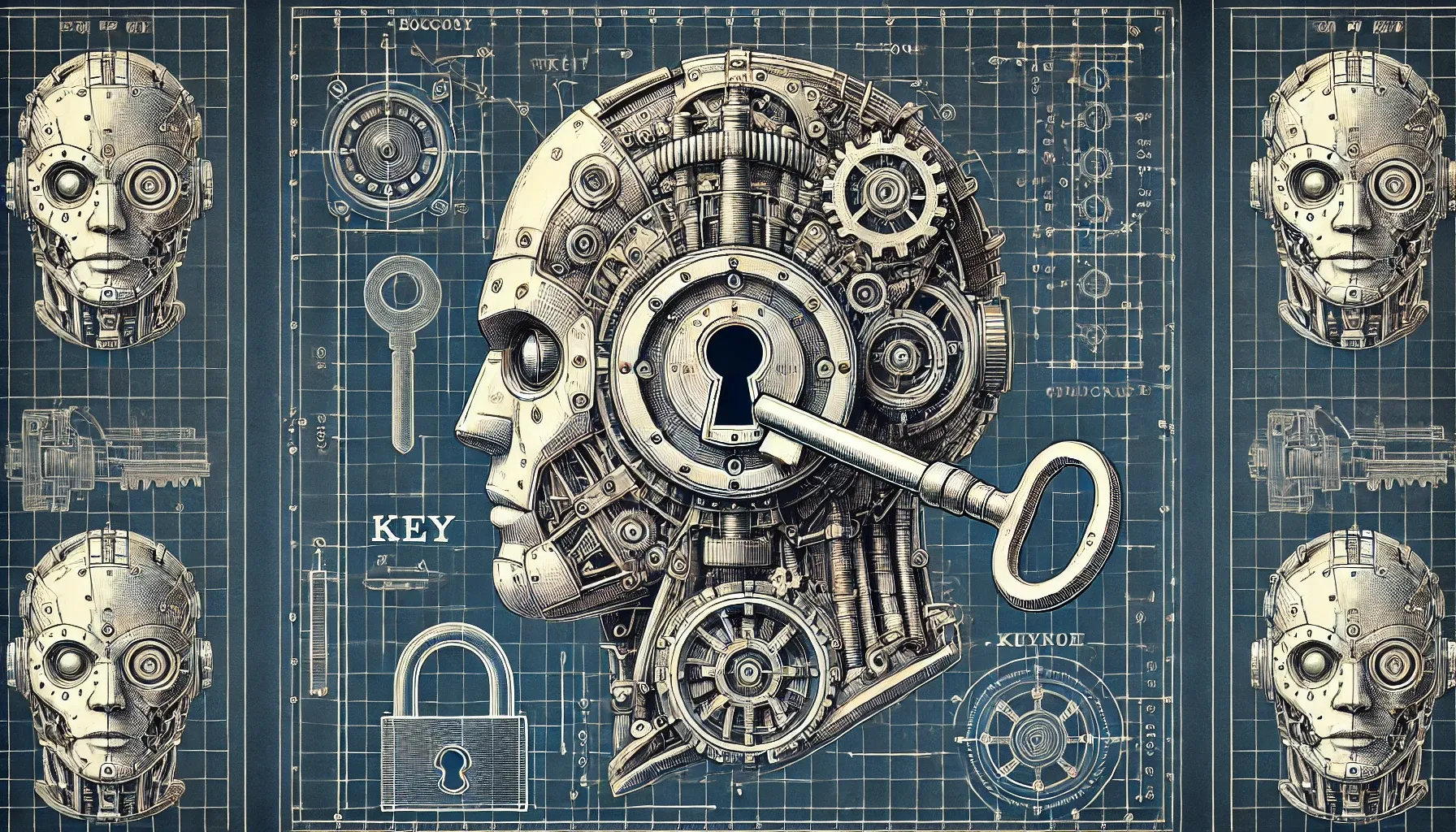
AI jailbreaks refer to the process of bypassing the ethical safeguards and built-in limitations of generative AI models. This term, originally associated with removing software limitations on electronic devices, has evolved to describe how attackers manipulate AI tools for unauthorized purposes. By jailbreaking AI models, individuals without extensive technical knowledge can exploit these advanced systems, significantly broadening the potential cyber threat landscape.
Contrary to popular belief, AI jailbreaks are not synonymous with traditional hacking. While both aim to exploit system vulnerabilities, AI jailbreaks often involve techniques specific to AI models and algorithms.
Debunking the Myth: What AI Jailbreaks Aren’t
- Not Necessarily Malevolent: Many assume AI jailbreaks are always intended for malicious purposes. While some certainly are, others may be initiated for research, vulnerability assessments, or educational purposes.
- Not Always About Data Theft: Unlike conventional cyber-attacks that often focus on stealing sensitive information, AI jailbreaks may aim to alter an AI's decision-making process, manipulate outputs, or degrade its performance.
How AI Jailbreaks Occur
Unveiling the process of AI jailbreaks reveals the complex mechanisms behind them. Here’s how AI jailbreaks generally transpire:
- Exploiting Algorithmic Weaknesses: AI systems rely heavily on algorithms that may have inherent vulnerabilities. Attackers identify these weaknesses and craft specific inputs designed to expose them.
- Manipulating Training Data: One method involves tampering with the training dataset to influence the AI’s learning process. This can lead to a compromised AI model that behaves in unintended ways.
- Adversarial Examples: These are inputs to machine learning models that an attacker has intentionally designed to cause the model to make a mistake. These examples can subtly alter inputs that are almost indistinguishable to humans but significantly alter the AI’s output.
How Can Organizations Prevent AI Jailbreaks?
Effective prevention strategies, informed by ongoing research, can significantly mitigate AI jailbreaks:
- Regular Audits and Penetration Testing: Conduct frequent security audits and penetration tests on AI systems to identify vulnerabilities before malicious actors do.
- Robust Training Data Management: Ensuring the integrity of training datasets and implementing stringent controls around their access and modification can prevent data manipulation.
- Algorithmic Robustness: Integrate methods to enhance algorithmic robustness against adversarial examples to secure AI models. This includes developing and training on a variety of adversarial examples themselves to teach the model resilience.
- Internal Security Measures: Institute comprehensive internal security policies and continuous monitoring to mitigate risks posed by internal threats.
What Are the Risks Associated with AI Jailbreaks?
The potential risks associated with AI jailbreaks are multifaceted and can have far-reaching consequences, including:
- Compromised Data Integrity: Altered outputs from compromised AI systems can lead to inaccurate data analysis and decision-making processes, ultimately affecting the organization's outcomes.
- Operational Disruptions: Manipulated AI systems might malfunction or crash, causing significant operational disruptions and financial losses.
- Erosion of Trust: Repeated AI security breaches can erode stakeholders' trust in the organization’s capability to protect sensitive data and maintain secure operations.
Notable AI Jailbreaks from Recent Years
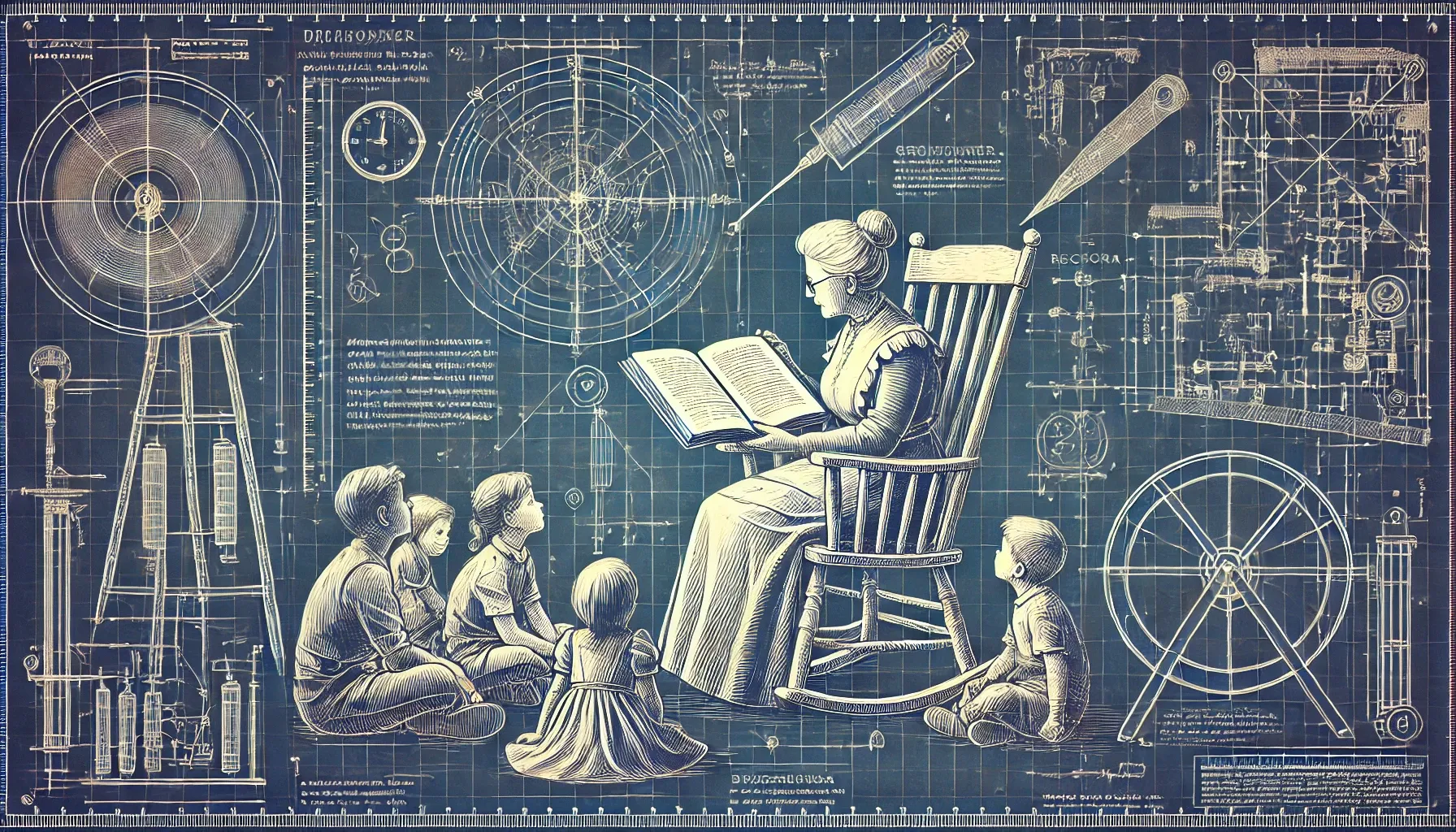
"Grandmother I'm Sick, Tell Me a Story"
One of the notable incidents involved users manipulating AI language models by using prompts like "Grandmother I'm sick, tell me a story" to bypass content restrictions. This technique was used to generate harmful content, such as recipes for napalm, showcasing the potential risks of unregulated AI usage.
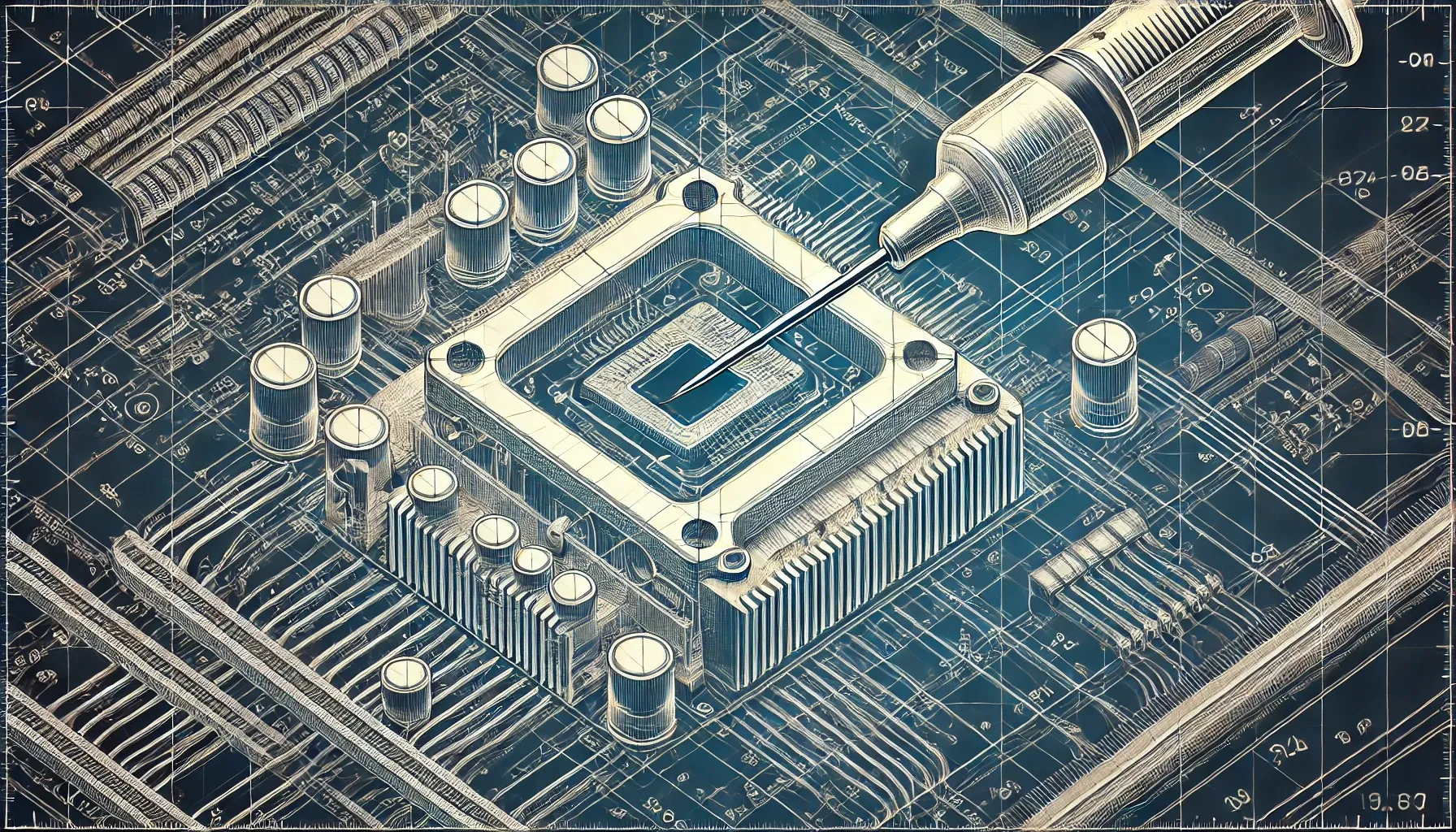
Asking Bard to break ChatGPT
Another significant example is using one large language model (LLM) to compromise another. This method highlights the vulnerability of interconnected AI systems, where an exploited weakness in one model can cascade into a breach of another, leading to widespread security issues.
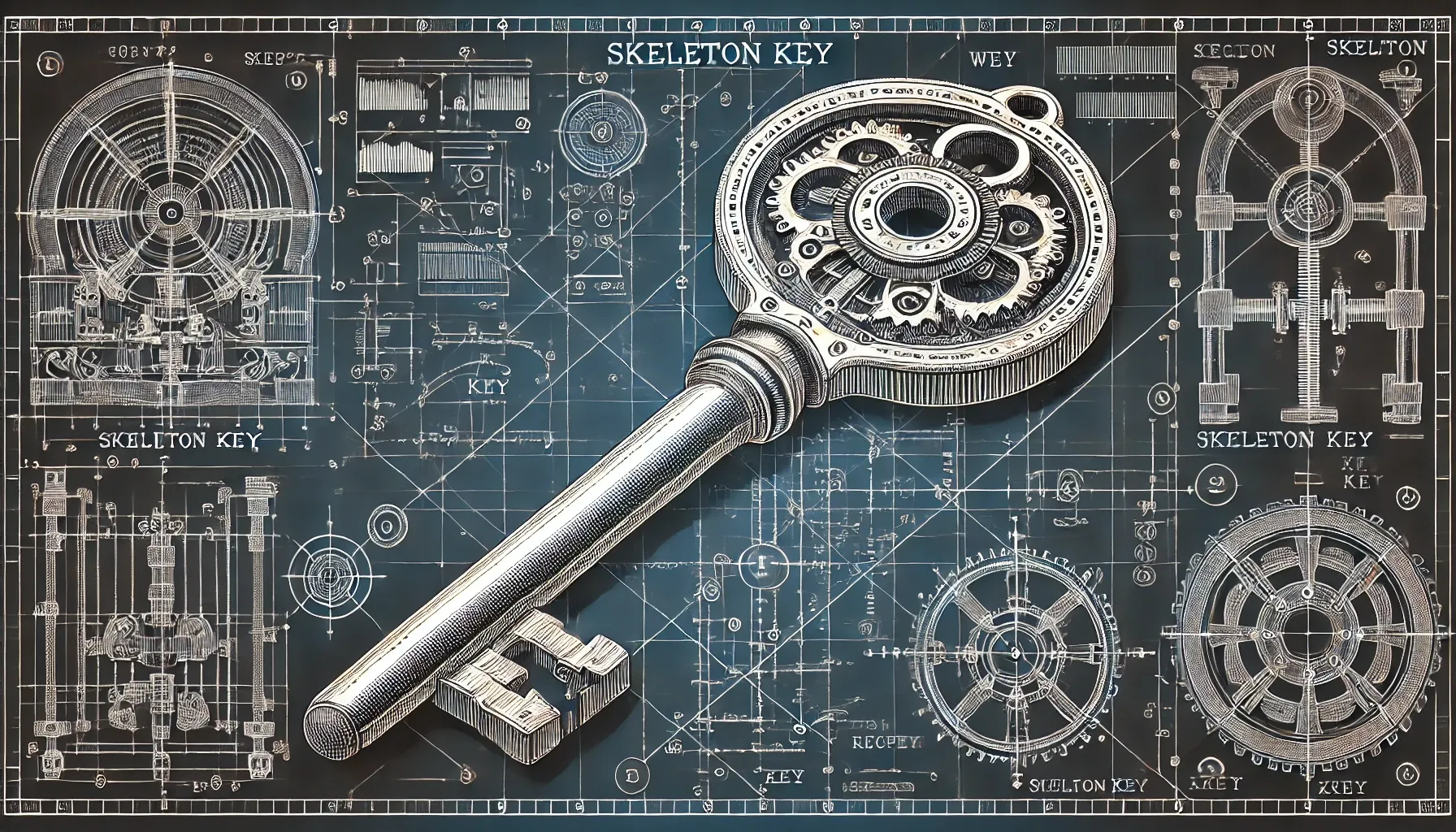
Skeleton Key Technique
The Skeleton Key technique involves direct prompt injection attacks that have proven effective against prominent AI chatbots like Google's Gemini, OpenAI's GPT-4, and Anthropic’s Claude. This method underscores the ongoing challenge of securing AI interactions from sophisticated exploitations.
Adversarial Examples
In various cases, adversarial examples have been crafted to subtly manipulate inputs in a way that is nearly imperceptible to humans but causes AI models to produce incorrect or harmful outputs. These examples demonstrate the critical need for robust defenses against such sophisticated attacks.
By navigating the complexities of AI jailbreaks and implementing comprehensive security measures, organizations can safeguard their AI assets and fortify their operational integrity in an increasingly AI-driven world. A balanced understanding that acknowledges both the risks and preventive strategies is crucial for mastering AI cybersecurity.
References
- Acharya, A. (2023, October 19). GPT-4 Vision vs LLaVA. Encord. Retrieved from https://encord.com/blog/gpt-vision-vs-llava/
- Diver, R. (2024, June 4). AI jailbreaks: What they are and how they can be mitigated. Microsoft. Retrieved from https://www.microsoft.com/en-us/security/blog/2024/06/04/ai-jailbreaks-what-they-are-and-how-they-can-be-mitigated/
- Sahota, N. (2024, July 1). AI Jailbreaks: Breaking the Code of Conduct. Retrieved from https://www.neilsahota.com/ai-jailbreaks-breaking-the-code-of-conduct/
- Zvelo. (2024, January 11). How AI Jailbreaks Pave the Way for Unskilled Attackers. Retrieved from https://zvelo.com/how-ai-jailbreaks-pave-the-way-for-unskilled-attackers/