Nvidia Blackwell by the numbers – the potential impact of Nvidia’s new AI superchip
NVIDIA CEO Jensen Huang recently described in detail the company’s latest AI accelerator chip, named Blackwell, at the company’s Computex 2024 keynote. With Blackwell, NVIDIA is aiming to cement its dominance in the burgeoning AI hardware space. With the company’s market cap racing towards the $3 trillion mark, NVIDIA’s rise to supreme command of AI infrastructure has been nothing short of astonishing. Huang sees no signs of progress stalling as the company continues to smash analyst expectations. But what do the specs and numbers really tell us about Blackwell’s capabilities and potential impact? Let’s take a closer look at how The post Nvidia Blackwell by the numbers – the potential impact of Nvidia’s new AI superchip appeared first on DailyAI.
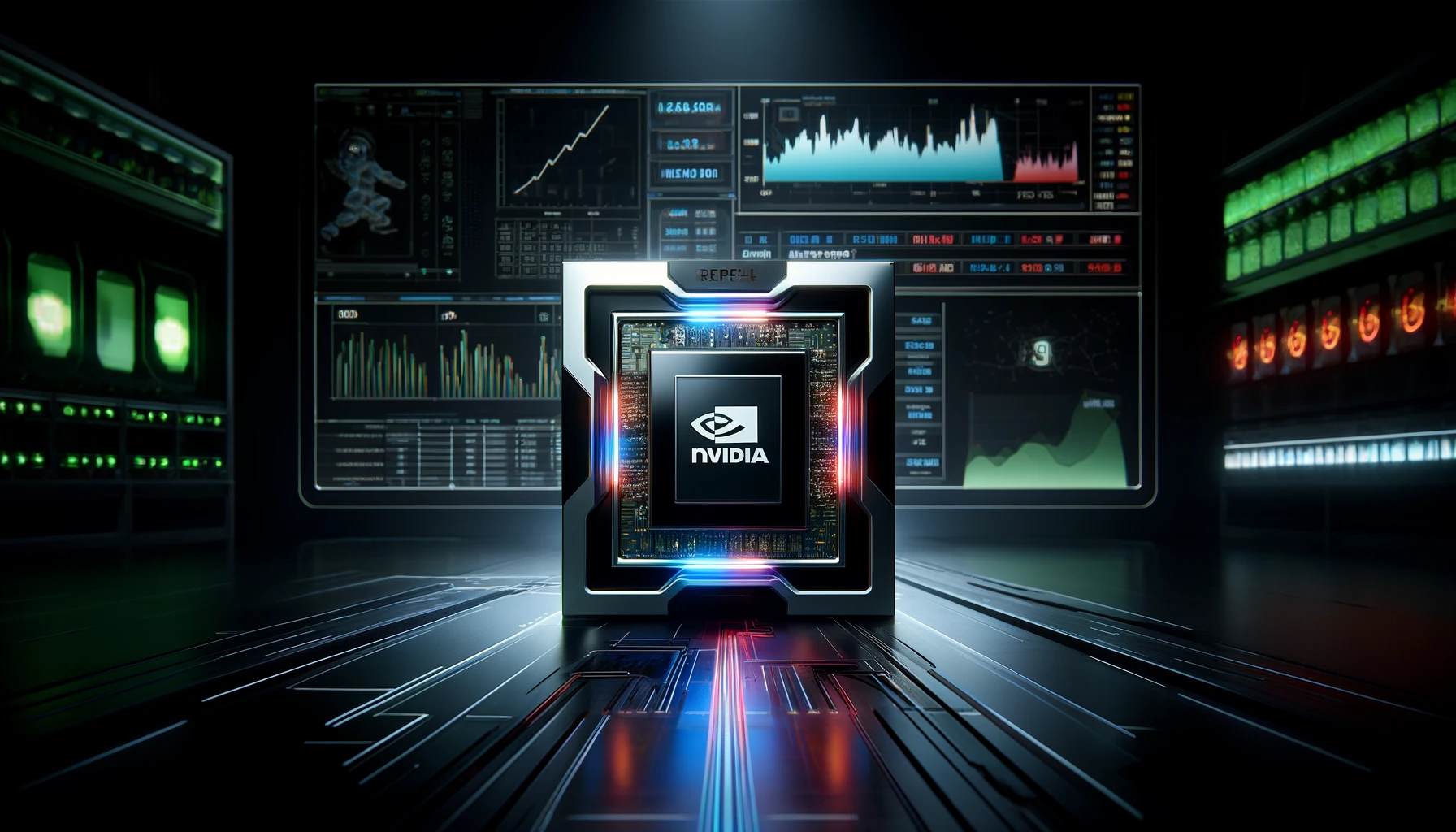
NVIDIA CEO Jensen Huang recently described in detail the company’s latest AI accelerator chip, named Blackwell, at the company’s Computex 2024 keynote.
With Blackwell, NVIDIA is aiming to cement its dominance in the burgeoning AI hardware space.
With the company’s market cap racing towards the $3 trillion mark, NVIDIA’s rise to supreme command of AI infrastructure has been nothing short of astonishing.
Huang sees no signs of progress stalling as the company continues to smash analyst expectations.
But what do the specs and numbers really tell us about Blackwell’s capabilities and potential impact?
Let’s take a closer look at how it might impact the AI industry and society at large.
Raw compute power
The headline figure is that a single Blackwell “superchip” – consisting of two GPU dies connected by a high-speed link – packs a whopping 208 billion transistors.
That’s nearly a 3X increase over NVIDIA’s previous generation Hopper chip. NVIDIA claims this translates to a 30X speed boost on AI inference tasks compared to Hopper.
To put that in perspective, let’s consider an example large language model with 100 billion parameters, similar in scale to GPT-3.
Training such a model on NVIDIA’s previous generation A100 GPUs would take around 1,024 A100 chips running for a month.
With Blackwell, NVIDIA claims that the same model could be trained in just over a week using 256 Blackwell chips – a 4X reduction in training time.
Energy efficiency
Despite its dramatic performance gains, NVIDIA states that Blackwell can reduce cost and energy consumption by up to 25X compared to Hopper for certain AI workloads.
The company provided the example of training a 1.8 trillion parameter model, which would have previously required 8,000 Hopper GPUs drawing 15 megawatts of power.
With Blackwell, NVIDIA says this could be accomplished with 2,000 GPUs drawing just 4 megawatts.
While a 4-megawatt power draw for a single AI training run is still substantial, it’s impressive that Blackwell can provide a nearly 4X boost in energy efficiency for such a demanding task.
Environmental costs
However, even with improved energy efficiency, widespread adoption of Blackwell could still significantly increase AI systems’ overall energy usage.
For example, let’s assume that there are currently 100,000 high-performance GPUs being used for AI training and inference worldwide.
If Blackwell enables a 10X increase in AI adoption over the forthcoming years, which doesn’t seem like an extraordinary figure to stick a pin in, that would mean 1 million Blackwell GPUs in use.
At the 1.875-kilowatt power draw per GPU that Huang cited, 1 million Blackwell GPUs would consume 1.875 gigawatts of power – nearly the output of two average nuclear power plants.
Previous analyses have forecasted that AI workloads might consume as much power as a small country by 2027.
Water consumption is also a massive issue, with Microsoft disclosing huge increases in their water consumption from 2022 to 2023, which correlated with AI model training and data center demand.
Without finding better ways to run AI hardware from renewables, the carbon emissions and water consumption from Blackwell-powered AI will be vast.
Beyond energy usage alone, it’s essential to consider other environmental costs, such as the rare earth minerals and other resources needed to manufacture advanced chips like Blackwell at scale and the waste generated when they reach end-of-life.
This isn’t to say that the societal benefits of the AI capabilities unlocked by Blackwell couldn’t outweigh these environmental costs, as Huang and other tech leaders are confident.
But it does mean that the environmental impact will need to be carefully managed and mitigated as part of any responsible Blackwell deployment plan, and there’s a lingering question mark over whether that’s possible.
Blackwell’s potential impact
So, what might the world look like in an era of widespread Blackwell adoption?
Some back-of-the-envelope estimates provide a sense of the possibilities and risks:
- Language models 10X the size of GPT-3 could be trained in a similar timeframe and using a similar amount of computing resources as GPT-3 did originally. This will enable a major leap in natural language AI capabilities.
- As described at the keynote, digital assistants with capabilities approaching humans could potentially become cost-effective in developing and deploying widely. An AI that could handle 80% of a typical knowledge work job’s tasks at 1/10th the cost of a human worker could displace up to 45 million jobs in the US alone.
- The computational capacity to train an AI system with general intelligence equal to or greater than the human brain may come within reach. Estimates for the brain’s computational capacity range from 10^13 to 10^16 neural connections. A Blackwell-powered supercomputer maxed out with 1 million GPUs would have an estimated 10^18 flops of compute – potentially sufficient to simulate aspects of the human brain in real-time.
Of course, these are highly speculative scenarios and should be taken with a large grain of salt. Technical feasibility doesn’t necessarily translate to real-world deployment.
They do, however, highlight the tremendous and disruptive potential of the AI acceleration NVIDIA is enabling with Blackwell.
Huang described Blackwell as “a new computing platform for a new computing era.” Based on the numbers, it’s hard to argue with that characterization.
Blackwell looks poised to usher in the next major phase of the AI revolution – for better or for worse.
As impressive as the chip’s specs are, society will need more than hardware innovations to grapple with the technology’s implications.
Careful consideration of the environmental impact and efforts to mitigate it must also be part of the equation.
While chips are becoming more energy-efficient, that alone is probably not enough to sustain current progress.
The post Nvidia Blackwell by the numbers – the potential impact of Nvidia’s new AI superchip appeared first on DailyAI.