How to collect voice data for machine learning
Machine learning and artificial intelligence have revolutionized our interactions with technology, mainly through speech recognition systems. At the core of these advancements lies voice data, a crucial component for training algorithms to understand and respond to human speech. The quality of this data significantly impacts the accuracy and efficiency of speech recognition models.Various industries, including automotive and healthcare, increasingly prioritize deploying responsive and reliable voice-operated systems.In this article, we’ll talk about the steps of voice data collection for machine learning. We’ll explore effective methods, address challenges, and highlight the essential role of high-quality data in enhancing speech recognition systems.Understanding the Challenges of Voice Data CollectionCollecting speech data for machine learning faces three key challenges. They impact the development and effectiveness of machine learning models. These challenges include:Varied Languages and AccentsGathering voice data across numerous languages and accents is a complex task. Speech recognition systems depend on this diversity to accurately comprehend and respond to different dialects. This diversity requires collecting a broad spectrum of data, posing a logistical and technical challenge.High CostAssembling a comprehensive voice dataset is expensive. It involves costs for recording, storage, and processing. The scale and diversity of data needed for effective machine learning further escalate these expenses.Lengthy TimelinesRecording and validating high-quality speech data is a time-intensive process. Ensuring its accuracy for effective machine learning models requires extended timelines for data collection.Data Quality and ReliabilityMaintaining the integrity and excellence of voice data is key to developing precise machine-learning models. This challenge involves meticulous data processing and verification.Technological LimitationsCurrent technology may limit the quality and scope of voice data collection. Overcoming these limitations is essential for developing advanced speech recognition systems.Methods of Collecting Voice DataYou have various methods available to collect voice data for machine learning. Each one comes with unique advantages and challenges.Prepackaged Voice DatasetsThese are ready-made datasets available for purchase. They offer a quick solution for basic speech recognition models and are typically of higher quality than public datasets. However, they may not cover specific use cases and require significant pre-processing.Public Voice DatasetsOften free and accessible, public voice datasets are useful for supporting innovation in speech recognition. However, they generally have lower quality and specificity than prepackaged datasets.Crowdsourcing Voice Data CollectionThis method involves collecting data through a wide network of contributors worldwide. It allows for customization and scalability in datasets. Crowdsourcing is cost-effective but may have equipment quality and background noise control limitations.Customer Voice Data CollectionGathering voice data directly from customers using products like smart home devices provides highly relevant and abundant data. This method raises ethical and privacy concerns. Thus, you might have to consider legal restrictions across certain regions.In-House Voice Data CollectionSuitable for confidential projects, this method offers control over the data collection, including device choice and background noise management. It tends to be costly and less diverse, and the real-time collection can delay project timelines.You may choose any method based on the project’s scope, privacy needs, and budget constraints.Exploring Innovative Use Cases and Sources for Voice DataVoice data is essential across various innovative applications.Conversational Agents: These agents, used in customer service and sales, rely on voice data to understand and respond to customer queries. Training them involves analyzing numerous voice interactions.Call Center Training: Voice data is crucial for training call center staff. It helps with accent correction and improves communication skills which enhance customer interaction quality.AI Content Creation: In content creation, voice data enables AI to produce engaging audio content. It includes podcasts and automated video narration.Smart Devices: Voice data is essential for smart home devices like virtual assistants and home automation systems. It helps these devices comprehend and execute voice commands accurately.Each of these use cases demonstrates the diverse applications of voice data in enhancing user experience and operational efficiency.Bridging Gaps and Ensuring Data QualityWe must actively diversify datasets to bridge gaps in voice data collection methodologies. This includes capturing a wider array of languages and accents. Such diversity ensures speech recognition systems perform effectively worldwide.Ensuring data qual
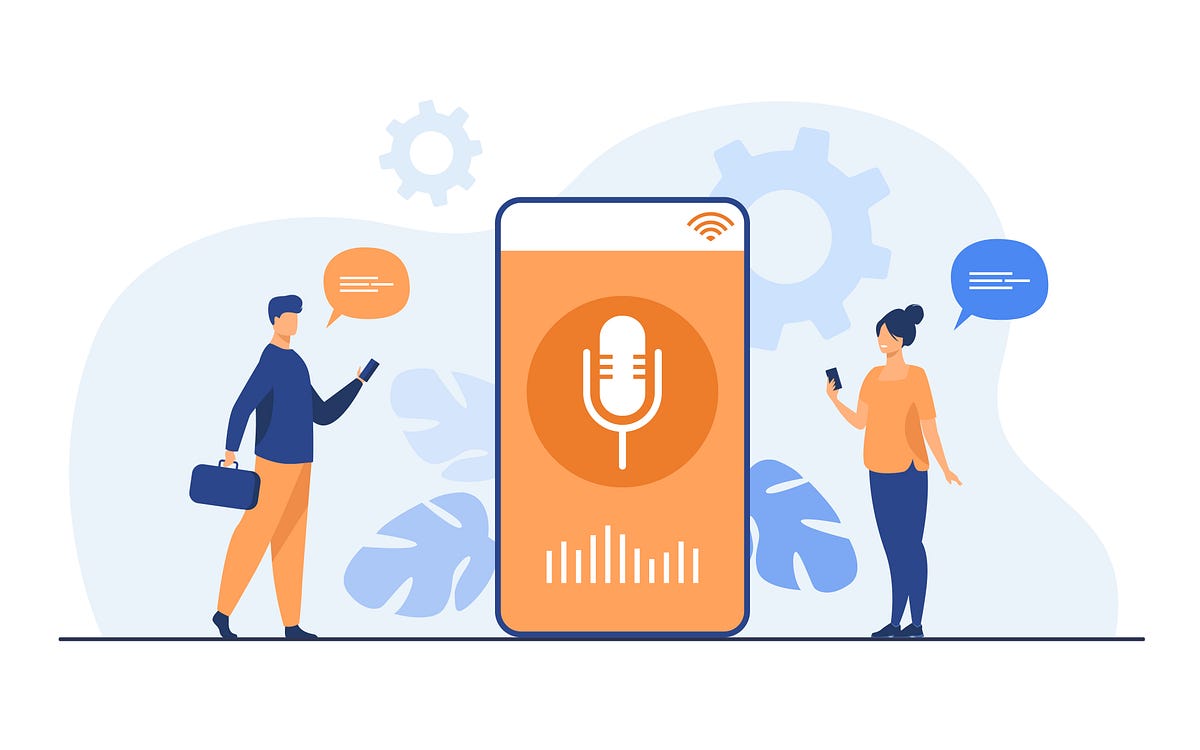
Machine learning and artificial intelligence have revolutionized our interactions with technology, mainly through speech recognition systems. At the core of these advancements lies voice data, a crucial component for training algorithms to understand and respond to human speech. The quality of this data significantly impacts the accuracy and efficiency of speech recognition models.
Various industries, including automotive and healthcare, increasingly prioritize deploying responsive and reliable voice-operated systems.
In this article, we’ll talk about the steps of voice data collection for machine learning. We’ll explore effective methods, address challenges, and highlight the essential role of high-quality data in enhancing speech recognition systems.

Understanding the Challenges of Voice Data Collection
Collecting speech data for machine learning faces three key challenges. They impact the development and effectiveness of machine learning models. These challenges include:
Varied Languages and Accents
Gathering voice data across numerous languages and accents is a complex task. Speech recognition systems depend on this diversity to accurately comprehend and respond to different dialects. This diversity requires collecting a broad spectrum of data, posing a logistical and technical challenge.
High Cost
Assembling a comprehensive voice dataset is expensive. It involves costs for recording, storage, and processing. The scale and diversity of data needed for effective machine learning further escalate these expenses.
Lengthy Timelines
Recording and validating high-quality speech data is a time-intensive process. Ensuring its accuracy for effective machine learning models requires extended timelines for data collection.
Data Quality and Reliability
Maintaining the integrity and excellence of voice data is key to developing precise machine-learning models. This challenge involves meticulous data processing and verification.
Technological Limitations
Current technology may limit the quality and scope of voice data collection. Overcoming these limitations is essential for developing advanced speech recognition systems.
Methods of Collecting Voice Data
You have various methods available to collect voice data for machine learning. Each one comes with unique advantages and challenges.
Prepackaged Voice Datasets
These are ready-made datasets available for purchase. They offer a quick solution for basic speech recognition models and are typically of higher quality than public datasets. However, they may not cover specific use cases and require significant pre-processing.
Public Voice Datasets
Often free and accessible, public voice datasets are useful for supporting innovation in speech recognition. However, they generally have lower quality and specificity than prepackaged datasets.
Crowdsourcing Voice Data Collection
This method involves collecting data through a wide network of contributors worldwide. It allows for customization and scalability in datasets. Crowdsourcing is cost-effective but may have equipment quality and background noise control limitations.
Customer Voice Data Collection
Gathering voice data directly from customers using products like smart home devices provides highly relevant and abundant data. This method raises ethical and privacy concerns. Thus, you might have to consider legal restrictions across certain regions.
In-House Voice Data Collection
Suitable for confidential projects, this method offers control over the data collection, including device choice and background noise management. It tends to be costly and less diverse, and the real-time collection can delay project timelines.
You may choose any method based on the project’s scope, privacy needs, and budget constraints.
Exploring Innovative Use Cases and Sources for Voice Data
Voice data is essential across various innovative applications.
- Conversational Agents: These agents, used in customer service and sales, rely on voice data to understand and respond to customer queries. Training them involves analyzing numerous voice interactions.
- Call Center Training: Voice data is crucial for training call center staff. It helps with accent correction and improves communication skills which enhance customer interaction quality.
- AI Content Creation: In content creation, voice data enables AI to produce engaging audio content. It includes podcasts and automated video narration.
- Smart Devices: Voice data is essential for smart home devices like virtual assistants and home automation systems. It helps these devices comprehend and execute voice commands accurately.
Each of these use cases demonstrates the diverse applications of voice data in enhancing user experience and operational efficiency.
Bridging Gaps and Ensuring Data Quality
We must actively diversify datasets to bridge gaps in voice data collection methodologies. This includes capturing a wider array of languages and accents. Such diversity ensures speech recognition systems perform effectively worldwide.
Ensuring data quality, especially in crowdsourced collections, is another key area. It demands improved verification methods for clarity and consistency. High-quality datasets are vital for different applications. They enable speech systems to understand varied speech patterns and nuances accurately.
Diverse and rich datasets are not just a technical necessity. They represent a commitment to inclusivity and global applicability in the evolving field of AI.
Ethical and Legal Considerations in Voice Data Collection
Ethical and legal considerations hold a lot of importance when collecting voice data, particularly from customers. These include:
- Privacy Concerns: Voice data is sensitive. Thus, you need to respect the user’s privacy.
- Consent: Obtaining explicit consent from individuals before collecting their voice data is a legal requirement in many jurisdictions.
- Transparency: Inform users about how you will use their data.
- Data Security: Implement robust measures to protect voice data from unauthorized access.
- Compliance with Laws: Adhere to relevant data protection laws, like GDPR, which govern the collection and use of personal data.
- Ethical Usage: Make sure you use the collected data ethically and do not harm individuals or groups.
Conclusion
The field of voice data collection for machine learning constantly evolves, facing new advancements and challenges. Key takeaways from this discussion include:
- Diverse Data Collection: Emphasize collecting varied languages and accents for global applicability.
- Cost-Benefit Analysis: Weigh the costs against the potential benefits of comprehensive datasets.
- Time Management: Plan for extended timelines due to the meticulous nature of data collection and validation.
- Legal and Ethical Compliance: Prioritize adherence to privacy laws and ethical standards.
- Quality Over Quantity: Focus on the quality and reliability of data for effective machine learning.
- Technological Adaptation: Stay updated with technological developments to enhance data collection methods.
These points show the dynamic nature of voice data collection. They highlight the need for innovative, ethical, and efficient approaches to machine learning.
How to collect voice data for machine learning was originally published in Becoming Human: Artificial Intelligence Magazine on Medium, where people are continuing the conversation by highlighting and responding to this story.