Here are the Applications of NLP in Finance. You Need to Know
Artificial intelligence, machine learning, natural language processing, and other related technologies are paving the way for a smarter “everything.” The integration of advanced technologies with finance provides better accuracy and data consistency across different operations.Where interpreting raw financial data has become easier NLP, it is also helping us make better predictions and financial decisions. NLP in finance includes semantic analysis, information extraction, and text analysis. As a result, we can automate manual processes, improve risk management, comply with regulations, and maintain data consistency. Going further, we will explore the benefits of natural language processing in finance and its use cases.How Does Data Labeling Work in Finance?Within NLP, data labeling allows machine learning models to isolate finance-related variables in different datasets. Using this training data, machine learning models can optimize data annotation, prediction, and analysis. Machine learning and artificial intelligence models need high-quality data to deliver the required results with higher accuracy and precision.However, to help these models provide optimized results, NLP labeling is essential. Financial data labeling with NLP is exercised with the following techniques;Sentiment analysis helps understand the sentiment behind investment decisions made by customers and investors.Document categorization includes sorting documents into groups for better classification and organization. The categories can be customized according to the data and requirements.Optical character recognition is a classification and organization NLP technique for document classification and digitization.Using these techniques, we can implement NLP for financial documents for effective data interpretation. Using this data, financial analysts and organizations can make informed decisions.Use Cases of NLP Data Labeling in FinanceLabeled data is used to train machine learning models, creating a better scope for supervised learning. As we get better data usability with NLP labeling, the number of applications increases.We generate tremendous amounts of financial data every day, and the vast majority of this data is unstructured. While analyzing this data is beneficial for the entire industry, doing so is a tedious task.To get useful information from this data, NLP models are deployed to analyze text and extract useful information. Financial organizations need accurate information to make better decisions for compliance and regulatory evaluation. With NLP, they can also stay updated with the changes in regulations and compliance requirements.Another application of NLP in finance is risk assessment, where organizations can determine the risk levels associated with a customer or entity based on their documentation and history. NLP can help declutter the information provided and extract information with NER and document categorization.Within this, the organizations can also use NLP risk models to automatically rank a customer’s credit profile to deliver a comprehensive analysis.Financial sentiment analysis is a bit different from regular sentiment analysis, even though both are performed with NLP. In the former, the analysis includes determining the market and customer reaction based on the stock price, market condition, a major event that can impact the markets, stocks, etc.Financial companies can use the information obtained to make better investment decisions and align their services with market conditions and sentiment.When banks and other financial institutions give out loans, they need to assess every profile for any sort of default risk or fraud. With NLP, organizations can fast-track this process as automated technologies help identify relevant information from a load of documents.NLP can easily analyze credit history, loan transactions, and income history with the motive to find and flag unusual activity. For this, the NLP techniques used are anomaly detection, sentiment annotation, classification, sequence annotation, and entity annotation.Financial organizations are also using NLP to make their accounts and auditing efficient. As NLP techniques can be used for documentation and text classification, this is beneficial for documenting reviews, checking procurement agreements, and other types of data.Within this, the organizations can also detect fraudulent activities and find traces of money laundering. As we employ NLP for financial documents, the techniques used include NER, sentiment analysis, topic modeling, and keyword extraction.Hidden between the vast amounts of data, NLP can find, identify, and extract relevant documents. As NLP technology and techniques use patterns to discover information, it is useful to process large amounts of unstructured data.The NLP techniques for this finance task include NER and Optical Character Recognition (OCR).The merger of ChatGPT and NLP in finance can provide better risk management and text-
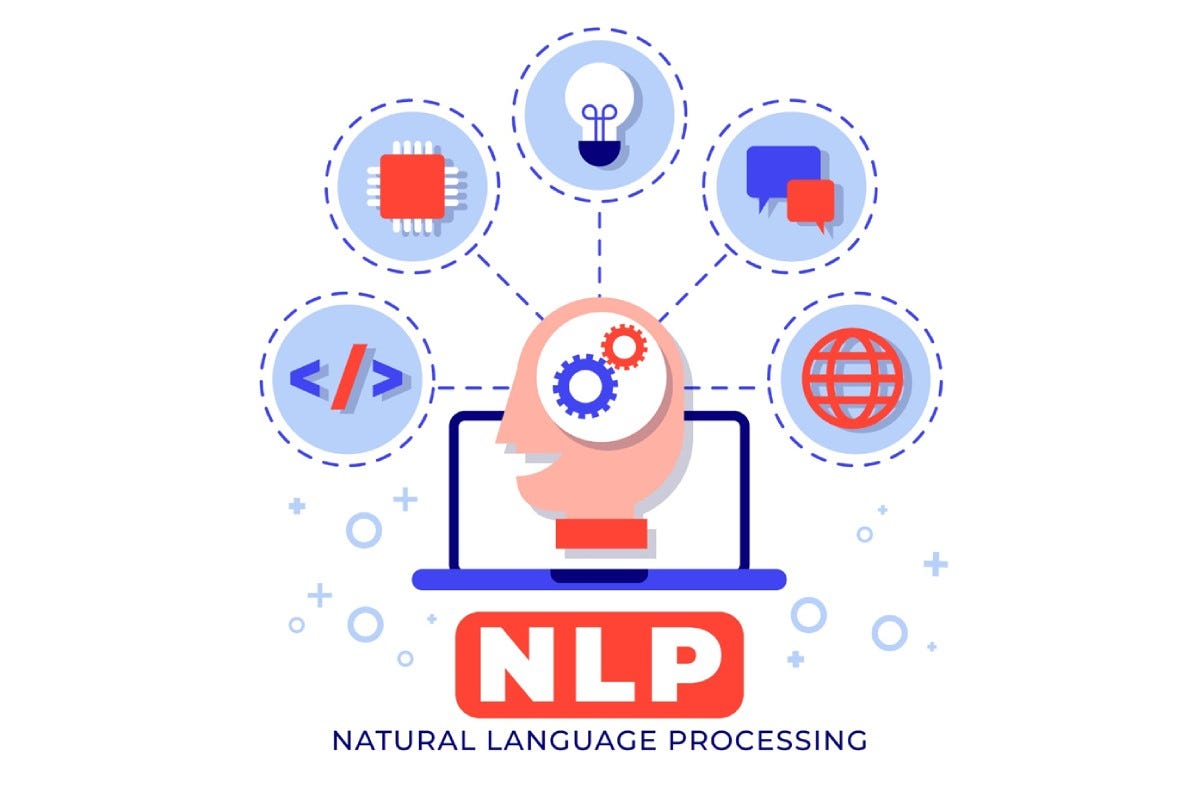
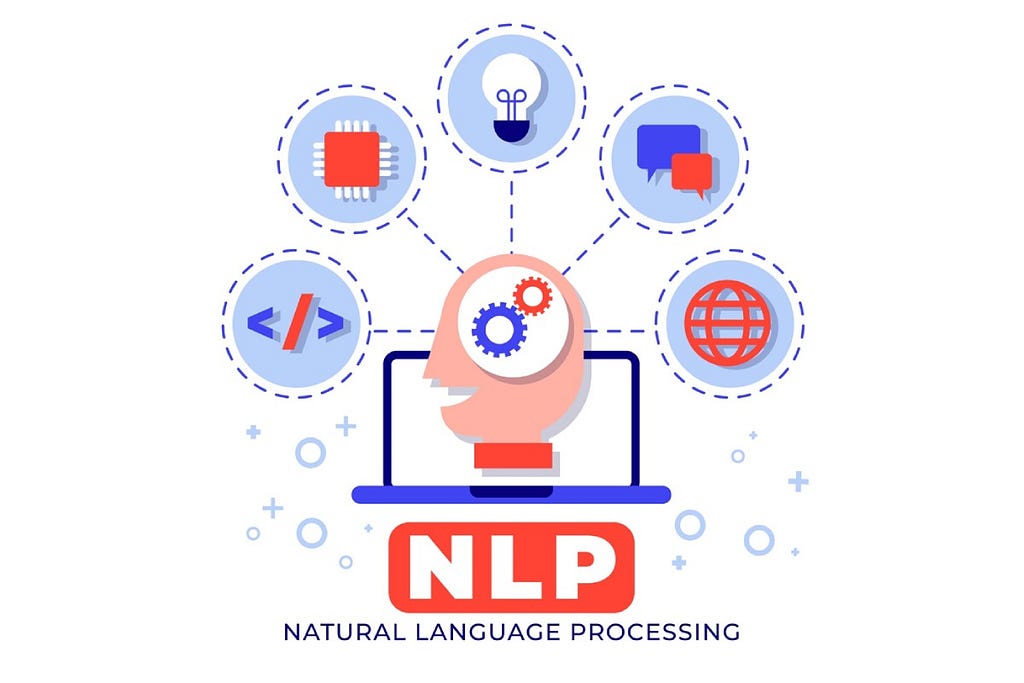
Artificial intelligence, machine learning, natural language processing, and other related technologies are paving the way for a smarter “everything.” The integration of advanced technologies with finance provides better accuracy and data consistency across different operations.
Where interpreting raw financial data has become easier NLP, it is also helping us make better predictions and financial decisions. NLP in finance includes semantic analysis, information extraction, and text analysis. As a result, we can automate manual processes, improve risk management, comply with regulations, and maintain data consistency. Going further, we will explore the benefits of natural language processing in finance and its use cases.
How Does Data Labeling Work in Finance?
Within NLP, data labeling allows machine learning models to isolate finance-related variables in different datasets. Using this training data, machine learning models can optimize data annotation, prediction, and analysis. Machine learning and artificial intelligence models need high-quality data to deliver the required results with higher accuracy and precision.
However, to help these models provide optimized results, NLP labeling is essential. Financial data labeling with NLP is exercised with the following techniques;
- Sentiment analysis helps understand the sentiment behind investment decisions made by customers and investors.
- Document categorization includes sorting documents into groups for better classification and organization. The categories can be customized according to the data and requirements.
- Optical character recognition is a classification and organization NLP technique for document classification and digitization.
Using these techniques, we can implement NLP for financial documents for effective data interpretation. Using this data, financial analysts and organizations can make informed decisions.
Use Cases of NLP Data Labeling in Finance
Labeled data is used to train machine learning models, creating a better scope for supervised learning. As we get better data usability with NLP labeling, the number of applications increases.
We generate tremendous amounts of financial data every day, and the vast majority of this data is unstructured. While analyzing this data is beneficial for the entire industry, doing so is a tedious task.
To get useful information from this data, NLP models are deployed to analyze text and extract useful information. Financial organizations need accurate information to make better decisions for compliance and regulatory evaluation. With NLP, they can also stay updated with the changes in regulations and compliance requirements.
Another application of NLP in finance is risk assessment, where organizations can determine the risk levels associated with a customer or entity based on their documentation and history. NLP can help declutter the information provided and extract information with NER and document categorization.
Within this, the organizations can also use NLP risk models to automatically rank a customer’s credit profile to deliver a comprehensive analysis.
Financial sentiment analysis is a bit different from regular sentiment analysis, even though both are performed with NLP. In the former, the analysis includes determining the market and customer reaction based on the stock price, market condition, a major event that can impact the markets, stocks, etc.
Financial companies can use the information obtained to make better investment decisions and align their services with market conditions and sentiment.
When banks and other financial institutions give out loans, they need to assess every profile for any sort of default risk or fraud. With NLP, organizations can fast-track this process as automated technologies help identify relevant information from a load of documents.
NLP can easily analyze credit history, loan transactions, and income history with the motive to find and flag unusual activity. For this, the NLP techniques used are anomaly detection, sentiment annotation, classification, sequence annotation, and entity annotation.
Financial organizations are also using NLP to make their accounts and auditing efficient. As NLP techniques can be used for documentation and text classification, this is beneficial for documenting reviews, checking procurement agreements, and other types of data.
Within this, the organizations can also detect fraudulent activities and find traces of money laundering. As we employ NLP for financial documents, the techniques used include NER, sentiment analysis, topic modeling, and keyword extraction.
Hidden between the vast amounts of data, NLP can find, identify, and extract relevant documents. As NLP technology and techniques use patterns to discover information, it is useful to process large amounts of unstructured data.
The NLP techniques for this finance task include NER and Optical Character Recognition (OCR).
The merger of ChatGPT and NLP in finance can provide better risk management and text-based financial analysis. Where GPT models are programmed with artificial intelligence and are meant to make our work productive and fast, ChatGPT can be deployed for in-depth text-based analysis.
In addition to analysis, it can be used for sentiment analysis, NER, and sentiment analysis. If that’s not all, we can also use ChatGPT to generate financial reports create summaries, and forecasts.
Conclusion
Natural language processing (NLP) has started an information extraction and analysis revolution in all industries. The versatility of this technology is also evolving to deliver better solutions and new applications. The usage of NLP in finance is not limited to the applications we have mentioned above. With time, we can use this technology and its techniques for even more complex tasks and operations.
As we expect the NLP technology to grow further and help with speech recognition, better data privacy, spam classification, etc. Shaip is at the forefront of understanding these applications and bringing them to your doorstep. We deliver intelligent NLP services aimed at delivering high-quality results to our clients. Better analysis and implementation will help you have a competitive advantage in the industry.
Author Bio
Vatsal Ghiya is a serial entrepreneur with more than 20 years of experience in healthcare AI software and services. He is the CEO and co-founder of Shaip, which enables the on-demand scaling of our platform, processes, and people for companies with the most demanding machine learning and artificial intelligence initiatives.Linkedin: https://www.linkedin.com/in/vatsal-ghiya-4191855/
Originally published at https://www.techiesguardian.com on October 13, 2023.
Here are the Applications of NLP in Finance. You Need to Know was originally published in Becoming Human: Artificial Intelligence Magazine on Medium, where people are continuing the conversation by highlighting and responding to this story.