University of Toronto researchers build peptide prediction model that beats AlphaFold 2
Scientists at the University of Toronto’s Donelly Centre have developed a cutting-edge AI model called PepFlow that can predict the diverse shapes that peptides adopt with unprecedented accuracy. Peptides are small molecules made up of amino acids, the building blocks of proteins. While peptides are similar to proteins, they’re much smaller and more flexible, allowing them to fold into a huge variety of shapes. A peptide’s specific shape is crucial because it determines how it interacts with other molecules in the body, which in turn dictates its biological function. Predicting the structures of proteins and peptides has been a longstanding The post University of Toronto researchers build peptide prediction model that beats AlphaFold 2 appeared first on DailyAI.
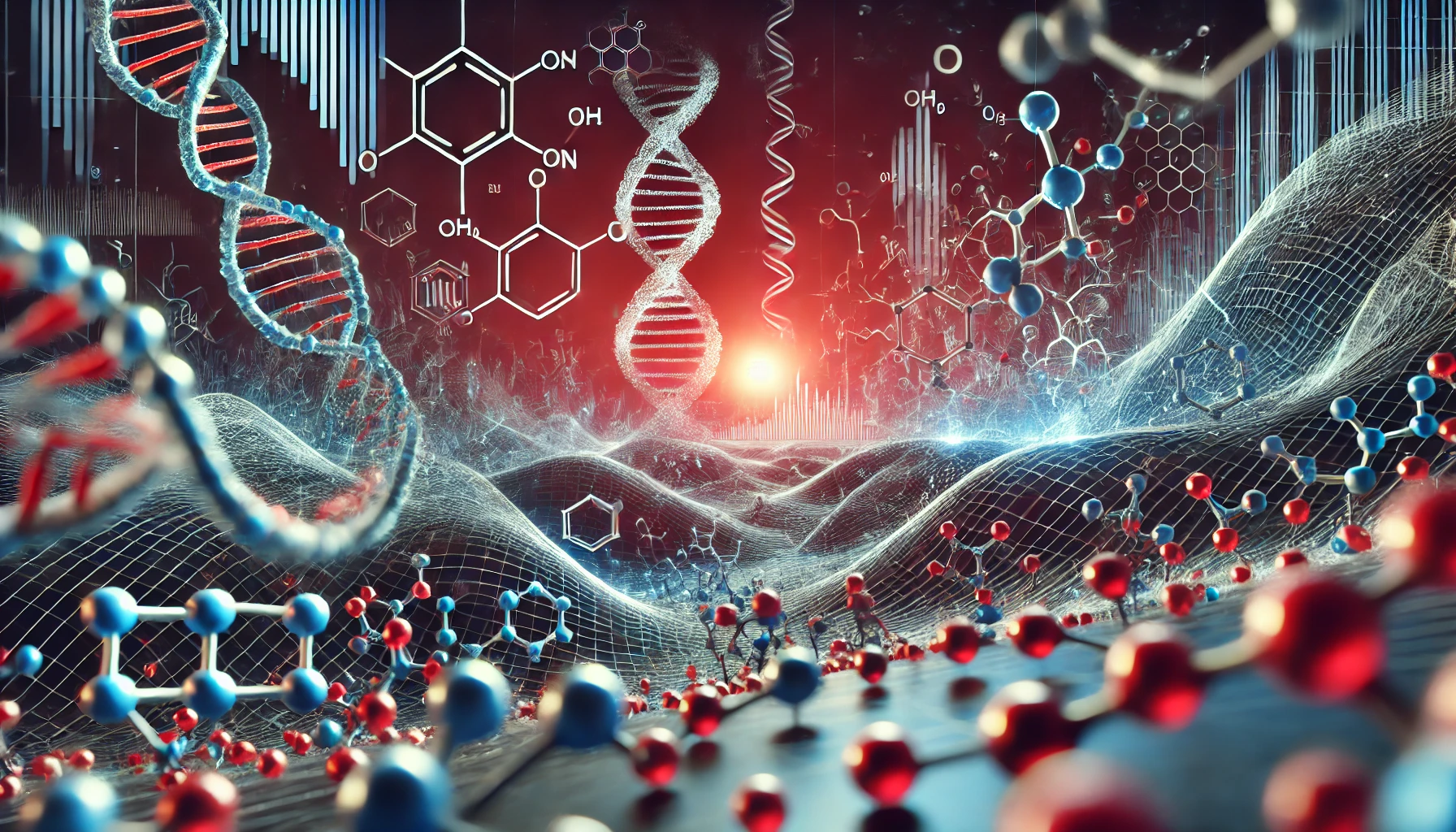
Scientists at the University of Toronto’s Donelly Centre have developed a cutting-edge AI model called PepFlow that can predict the diverse shapes that peptides adopt with unprecedented accuracy.
Peptides are small molecules made up of amino acids, the building blocks of proteins.
While peptides are similar to proteins, they’re much smaller and more flexible, allowing them to fold into a huge variety of shapes.
A peptide’s specific shape is crucial because it determines how it interacts with other molecules in the body, which in turn dictates its biological function.
Predicting the structures of proteins and peptides has been a longstanding challenge in biology. Due to the complex math involved, it’s an excellent problem for machine learning.
In recent years, AI models like AlphaFold2 and 3, developed by Google’s DeepMind, have revolutionized protein structure prediction.
AlphaFold2 uses deep learning to predict a protein’s most likely 3D structure based on its amino acid sequence. But while AlphaFold2 has been incredibly successful for proteins, it has limitations when it comes to highly flexible molecules like peptides.
“We haven’t been able to model the full range of conformations for peptides until now,” said Osama Abdin, the study’s first author.
Pepflow, documented in a study published in Nature Machine Intelligence, “leverages deep-learning to capture the precise and accurate conformations of a peptide within minutes.”
PepFlow employs AI models inspired by Boltzmann generators. These models learn the fundamental physical principles that govern how a peptide’s chemical structure determines its spectrum of possible shapes.
This allows PepFlow to accurately predict the structures of peptides with unusual features, such as circular peptides formed through macrocyclization. Macrocyclic peptides are particularly interesting for drug development due to their unique binding properties.
What sets PepFlow apart from models like AlphaFold2 is its ability to predict not just one structure, but the entire “energy landscape” of a peptide.
The energy landscape represents all the possible shapes a peptide can take and how it transitions between these different conformations.
Capturing this structural complexity is key to understanding how peptides function in different biological contexts.
Researchers at @UofT have developed a #DeepLearning model, called PepFlow, that can predict all possible shapes of peptides.
PepFlow can inform drug development through the design of peptides that act as binders. #DrugDiscovery
Learn more
https://t.co/eAKOg5e7Cz pic.twitter.com/mYP9YeiCOe
— Donnelly Centre (@DonnellyCentre) June 27, 2024
Significance
The ability to predict highly accurate peptide structures has major implications for developing peptide-based therapeutics.
“Peptides were the focus of the PepFlow model because they are very important biological molecules and they are naturally very dynamic, so we need to model their different conformations to understand their function,” explained Philip M. Kim, the study’s lead investigator.
“They’re also important as therapeutics, as can be seen by the GLP1 analogues, like Ozempic, used to treat diabetes and obesity.”
Peptide drugs have several advantages over traditional small-molecule drugs and larger protein-based therapeutics. They’re more specific in their actions, have lower toxicity than small-molecule drugs, and are cheaper and easier to produce than larger protein drugs.
PepFlow could accelerate the discovery and development of new peptide-based medicines by enabling the design of peptides with therapeutic properties.
“Modelling with PepFlow offers insight into the real energy landscape of peptides,” said Abdin.
“It took two-and-a-half years to develop PepFlow and one month to train it, but it was worthwhile to move to the next frontier, beyond models that only predict one structure of a peptide.”
The post University of Toronto researchers build peptide prediction model that beats AlphaFold 2 appeared first on DailyAI.