The future of AI training: DisTrO’s game-changing approach
Applied AI research group Nous Research developed an AI model training optimizer that could dramatically change the way AI models of the future will be trained. Traditionally, training an AI model requires massive data centers packed with GPUs like NVIDIA’s H100s, and high-speed interconnects to synchronize gradient and parameter updates between GPUs. Each training step requires vast amounts of data to be shared between thousands of GPUs. The required bandwidth means these GPUs need to be hardwired and physically close to each other. With DisTrO, Nous Research may have found a way to change that completely. As a model is The post The future of AI training: DisTrO’s game-changing approach appeared first on DailyAI.
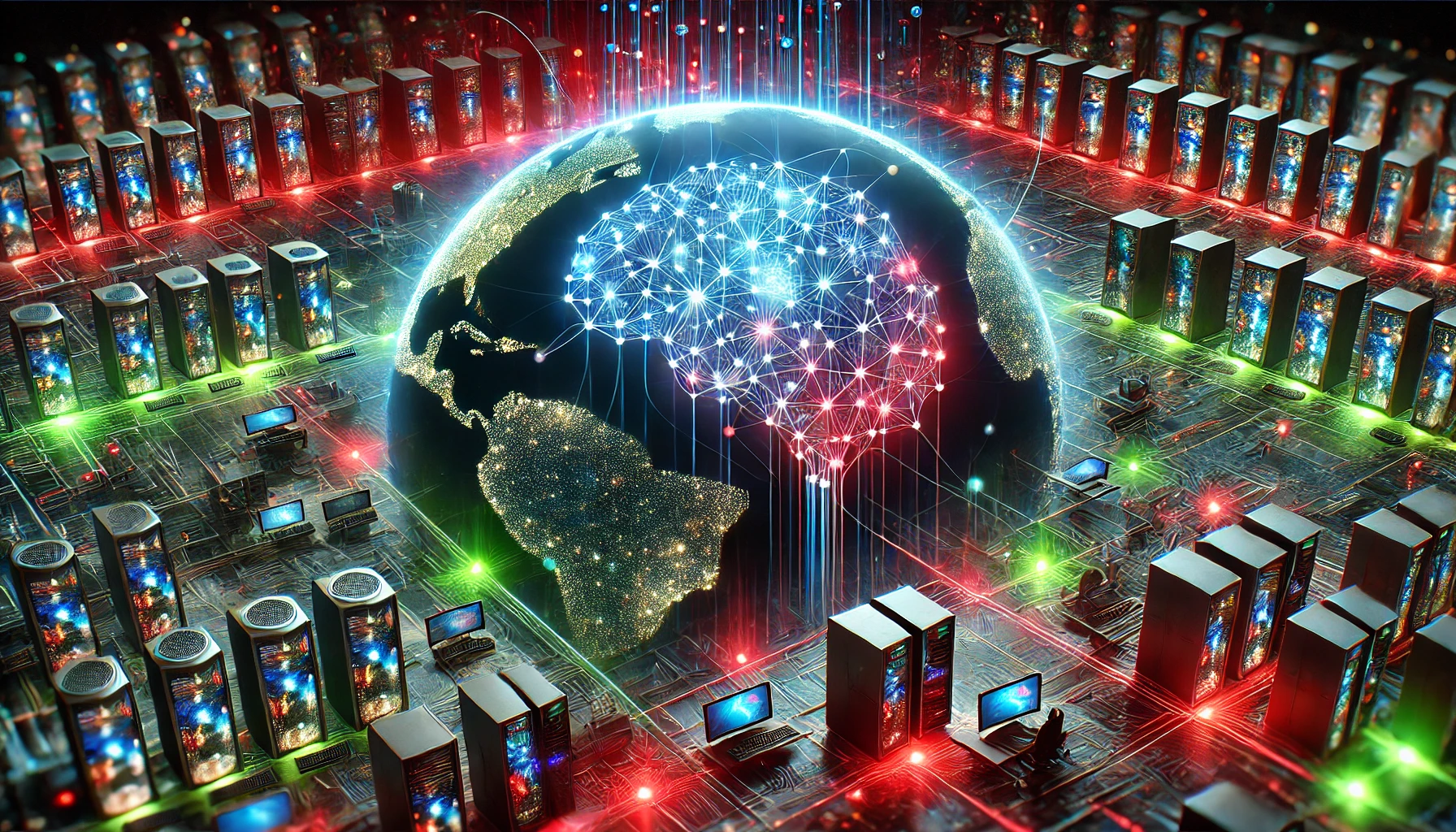
Applied AI research group Nous Research developed an AI model training optimizer that could dramatically change the way AI models of the future will be trained.
Traditionally, training an AI model requires massive data centers packed with GPUs like NVIDIA’s H100s, and high-speed interconnects to synchronize gradient and parameter updates between GPUs.
Each training step requires vast amounts of data to be shared between thousands of GPUs. The required bandwidth means these GPUs need to be hardwired and physically close to each other. With DisTrO, Nous Research may have found a way to change that completely.
As a model is trained, an optimizer algorithm adjusts the parameters of the model to minimize the loss function. The loss function measures the difference between the model’s predictions and the actual outcomes, and the goal is to reduce this loss as much as possible through iterative training.
DisTrO-AdamW is a variation of the popular AdamW optimizer algorithm. DisTrO stands for “Distributed Training Over-the-Internet” and hints at what makes it so special.
DisTrO-AdamW drastically reduces the amount of inter-GPU communication required during the training of large neural networks. And it does this without sacrificing the convergence rate or accuracy of the training process.
In empirical tests, DisTrO-AdamW achieved an 857x reduction in inter-GPU communication. This means that the DisTrO approach can train models with comparable accuracy and speed but without the need for expensive, high-bandwidth hardware.
For example, during the pre-training of a 1.2 billion LLM, DisTrO-AdamW matched the performance of traditional methods while reducing the required bandwidth from 74.4 GB to just 86.8 MB per training step.
What if you could use all the computing power in the world to train a shared, open source AI model?
Preliminary report: https://t.co/b1XgJylsnV
Nous Research is proud to release a preliminary report on DisTrO (Distributed Training Over-the-Internet) a family of… pic.twitter.com/h2gQJ4m7lB
— Nous Research (@NousResearch) August 26, 2024
Implications for AI Training
DisTrO’s impact on the AI landscape could be profound. By reducing the communication overhead, DisTrO allows for the decentralized training of large models. Instead of a data center with thousands of GPUs and high-speed switches, you could train a model on distributed commercial hardware connected via the internet.
You could have a community of people contributing access to their computing hardware to train a model. Imagine millions of idle PCs or redundant Bitcoin mining rigs working together to train an open source model. DisTrO makes that possible, and there’s hardly any sacrifice in the time to train the model or its accuracy.
Nous Research admits they’re not really sure why their approach works so well and more research is needed to see if it scales to larger models.
If it does, training massive models might no longer be monopolized by Big Tech companies with the cash needed for large data centers. It could also have a big impact by reducing the environmental impact of energy and water-hungry data centers.
The concept of decentralized training could also make some aspects of regulations like California’s proposed SB 1047 bill moot. The bill calls for additional safety checks for models that cost more than $100m to train.
With DisTrO, a community of anonymous people with distributed hardware could create a ‘supercomputer’ of their own to train a model. It could also negate the US government’s efforts to stop China from importing NVIDIA’s most powerful GPUs.
In a world where AI is becoming increasingly important, DisTrO offers a glimpse of a future where the development of these powerful tools is more inclusive, sustainable, and widespread.
The post The future of AI training: DisTrO’s game-changing approach appeared first on DailyAI.