Is “fear” the key to building more adaptable, resilient, and natural AI systems?
AI research is fueled by the pursuit of ever-greater sophistication, which includes training systems to think and behave like humans. The end goal? Who knows. The goal for now? To create autonomous, generalized AI agents capable of performing a wide range of tasks. This concept is called artificial general intelligence (AGI) or superintelligence, which means the same thing. It’s challenging to pinpoint precisely what AGI entails because there’s virtually zero consensus on what ‘intelligence’ is, or indeed, when or how artificial systems might achieve it. Some even believe AI, in its current state, can never truly obtain general intelligence. Professor The post Is “fear” the key to building more adaptable, resilient, and natural AI systems? appeared first on DailyAI.
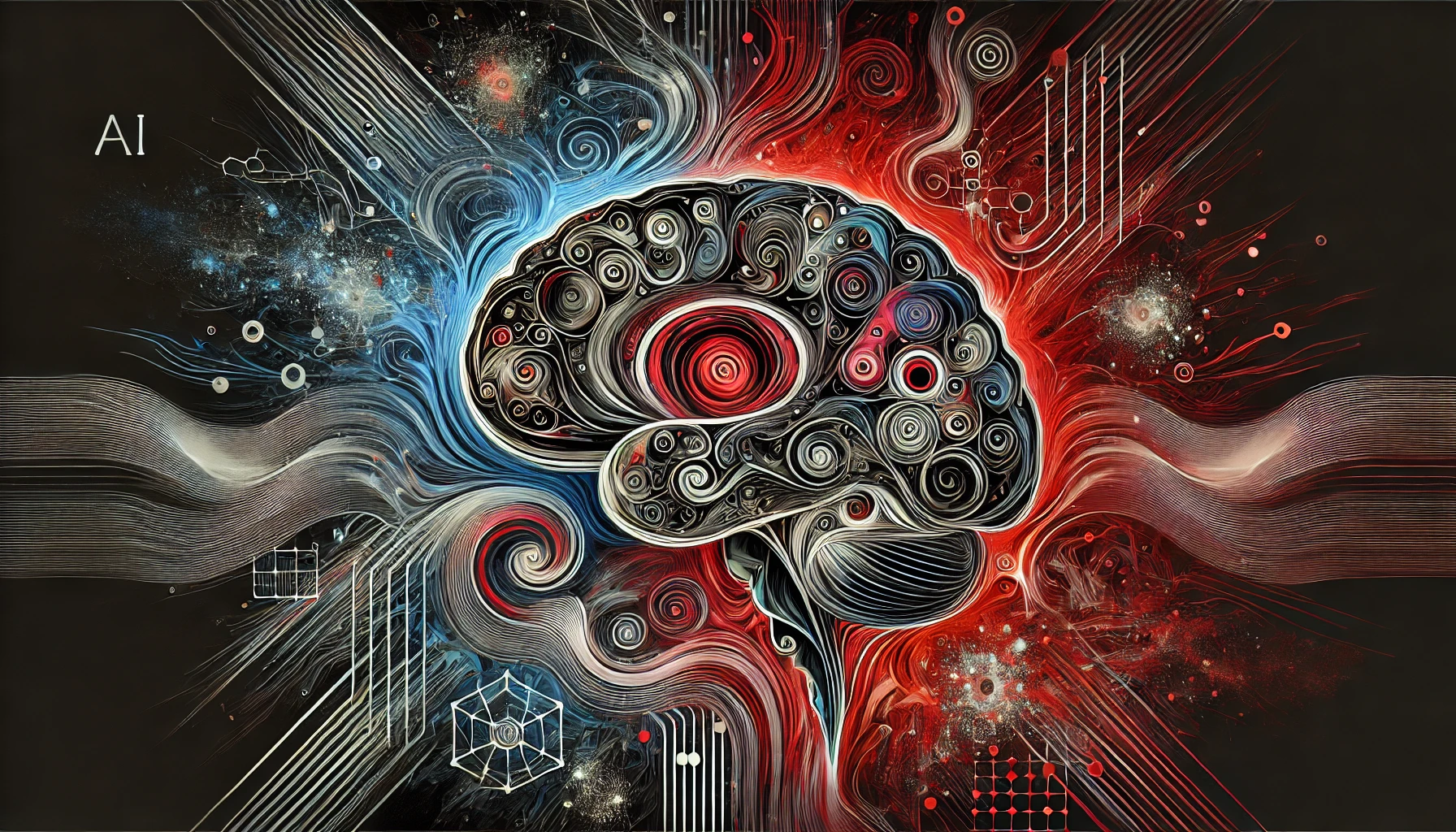
AI research is fueled by the pursuit of ever-greater sophistication, which includes training systems to think and behave like humans.
The end goal? Who knows. The goal for now? To create autonomous, generalized AI agents capable of performing a wide range of tasks.
This concept is called artificial general intelligence (AGI) or superintelligence, which means the same thing.
It’s challenging to pinpoint precisely what AGI entails because there’s virtually zero consensus on what ‘intelligence’ is, or indeed, when or how artificial systems might achieve it.
Some even believe AI, in its current state, can never truly obtain general intelligence.
Professor Tony Prescott and Dr. Stuart Wilson from the University of Sheffield described generative language models, like ChatGPT, as inherently limited because they’re “disembodied” and have no sensory perception or grounding in the natural world.
Meta’s chief AI scientist, Yann LeCun, said even a house cat’s intelligence is fathomlessly more advanced than today’s best AI systems.
“But why aren’t those systems as smart as a cat?” LeCun asked at the World Government Summit in Dubai.
“A cat can remember, can understand the physical world, can plan complex actions, can do some level of reasoning—actually much better than the biggest LLMs. That tells you we are missing something conceptually big to get machines to be as intelligent as animals and humans.”
While these skills may not be necessary to achieve AGI, there is some consensus that moving complex AI systems from the lab into the real world will require adopting behaviors similar to those observed in natural organisms.
So, how can this be achieved? One approach is to dissect elements of cognition into basic components and work out how AI systems can mimic them.
A previous DailyAI essay investigated curiosity and its ability to guide organisms toward new experiences and objectives, fueling the collective evolution of the natural world.
But there is another emotion – another essential component of our existence – from which AGI could benefit. And that’s fear.
How AI can learn from biological fear
Far from being a weakness or a flaw, fear is one of evolution’s most potent tools for keeping organisms safe.
The amygdala is the central structure that governs fear in vertebrates. In humans, it’s a small, almond-shaped structure nestled deep within the brain’s temporal lobes.
Often dubbed the “fear center,” the amygdala serves as an early warning system, constantly scanning incoming sensory information for potential threats.
When a threat is detected – whether it’s the sudden lurch of a braking car ahead or a shifting shadow in the darkness – the amygdala springs into action, triggering a cascade of physiological and behavioral changes optimized for rapid defensive response:
- Heart rate and blood pressure surge, priming the body for “fight or flight”
- Attention narrows and sharpens, honing in on the source of danger
- Reflexes quicken, readying muscles for split-second evasive action
- Cognitive processing shifts to a rapid, intuitive, “better safe than sorry” mode
This response is not a simple reflex but a highly adaptive, context-sensitive suite of changes that flexibly tailor behavior to the nature and severity of the threat at hand.
It’s also exceptionally quick. We become consciously aware of a threat around 300-400 milliseconds after initial detection.
Moreover, the amygdala doesn’t operate in isolation. It’s densely interconnected with other key brain regions involved in perception, memory, reasoning, and action.
Why fear might benefit AI
So, why does fear matter in the context of AI anyway?
In biological systems, fear serves as a crucial mechanism for rapid threat detection and response. By mimicking this system in AI, we can potentially create more robust and adaptable artificial systems.
This is particularly pertinent to autonomous systems that interact with the real world. Case in point: despite AI intelligence exploding in recent years, driverless cars still tend to fall short in safety and reliability.
Regulators are probing numerous fatal incidents involving self-driving cars, including Tesla models with Autopilot and Full Self-Driving features.
Speaking to the Guardian in 2022, Matthew Avery, director of research at Thatcham Research, explained why driverless cars have been so challenging to refine:
“Number one is that this stuff is harder than manufacturers realized,” Avery states.
Avery estimates that around 80% of autonomous driving functions involve relatively straightforward tasks like lane following and basic obstacle avoidance.
The next actions, however, are much more challenging. “The last 10% is really difficult,” Avery emphasizes, like “when you’ve got, you know, a cow standing in the middle of the road that doesn’t want to move.”
Sure, cows aren’t fear-inspiring in their own right. But any concentrating driver would probably fancy their chances at stopping if they’re hurtling towards one at speed.
An AI system relies on its training and technology to see the cow and make the appropriate decision. That process isn’t always quick or reliable enough, hence a high risk of collisions and accidents, especially when the system encounters something it’s not trained to understand.
Imbuing AI systems with fear might provide an alternative, quicker, and more efficient means of reaching that decision.
In biological systems, fear triggers rapid, instinctive responses that don’t require complex processing. For instance, a human driver might instinctively brake at the mere suggestion of an obstacle, even before fully processing what it is.
This near-instantaneous reaction, driven by a fear response, could be the difference between a near-miss and a collision.
Moreover, fear-based responses in nature are highly adaptable and generalize well to novel situations.
An AI system with a fear-like mechanism could be better equipped to handle unforeseen scenarios.
Deconstructing fear: insights from the fruit fly
We’re far from developing artificial systems that replicate the integrated, specialized neural regions in biological brains, but that doesn’t mean we can’t model those mechanisms in other ways.
So, let’s zoom out from the amygdala and look at how invertebrates – small insects, for example – detect and process fear.
While they don’t have a structure directly analogous to the amygdala, that doesn’t mean they lack circuitry that achieves a similar objective.
For example, recent studies into the fear responses of Drosophila melanogaster, the common fruit fly, yielded intriguing insights into the fundamental building blocks of primitive emotion.
In an experiment conducted at Caltech in 2015, researchers led by David Anderson exposed flies to an overhead shadow designed to mimic an approaching predator.
Using high-speed cameras and machine vision algorithms, they meticulously analyzed the flies’ behavior, looking for signs of what Anderson calls “emotion primitives” – the basic components of an emotional state.
Remarkably, the flies exhibited a suite of behaviors that closely paralleled the fear responses seen in mammals.
When the shadow appeared, the flies froze in place, and their wings cocked at an angle to prepare for a quick escape.
As the threat persisted, some flies took flight, darting away from the shadow at high speed. Others remained frozen for an extended period, suggesting a state of heightened arousal and vigilance.
Crucially, these responses were not mere reflexes triggered automatically by the visual stimulus. Instead, they appeared to reflect an enduring internal state, a kind of “fly fear” that persisted even after the threat had passed.
This was evident in the fact that the flies’ heightened defensive behaviors could be elicited by a different stimulus (a puff of air) even minutes after the initial shadow exposure.
Moreover, the intensity and duration of the fear response scaled with the level of threat. Flies exposed to multiple shadow presentations showed progressively stronger and longer-lasting defensive behaviors, indicating a kind of “fear learning” that allowed them to calibrate their response based on the severity and frequency of the danger.
As Anderson and his team argue, these findings suggest that the building blocks of emotional states – persistence, scalability, and generalization – are present even in the simplest creatures.
If we can decode how simpler organisms like fruit flies process and respond to threats, we can potentially extract the core principles of adaptive, self-preserving behavior.
Primitive forms of fear could be applied to develop AI systems that are more robust, safer, and attuned to real-world risks and challenges.
Infusing AI with fear circuitry
It’s a great theory, but can AI be imbued with an authentic, functional form of ‘fear’ in practice?
One intriguing study examined exactly that with the aim of improving the safety of driverless cars and other autonomous systems.
“Fear-Neuro-Inspired Reinforcement Learning for Safe Autonomous Driving,” led by Chen Lv at Nanyang Technological University, Singapore, developed a fear-neuro-inspired reinforcement learning (FNI-RL) framework for improving the performance of driverless cars.
By building AI systems that can recognize and respond to the subtle cues and patterns that trigger human defensive driving – what they term “fear neurons” – we may be able to create self-driving cars that navigate the road with the intuitive caution and risk sensitivity they need.
The FNI-RL framework translates key principles of the brain’s fear circuitry into a computational model of threat-sensitive driving, allowing an autonomous vehicle to learn and deploy adaptive defensive strategies in real time.
It involves three key components modeled after core elements of the neural fear response:
- A “fear model” that learns to recognize and assess driving situations that signal heightened collision risk, playing a role analogous to the threat-detection functions of the amygdala.
- An “adversarial imagination” module that mentally simulates dangerous scenarios, allowing the system to safely “practice” defensive maneuvers without real-world consequences – a form of risk-free learning reminiscent of the mental rehearsal capacities of human drivers.
- A “fear-constrained” decision-making engine that weighs potential actions not just by their immediately expected rewards (e.g. progress towards a destination), but also by their assessed level of risk as gauged by the fear model and adversarial imagination components. This mirrors the amygdala’s role in flexibly guiding behavior based on an ongoing calculus of threat and safety.
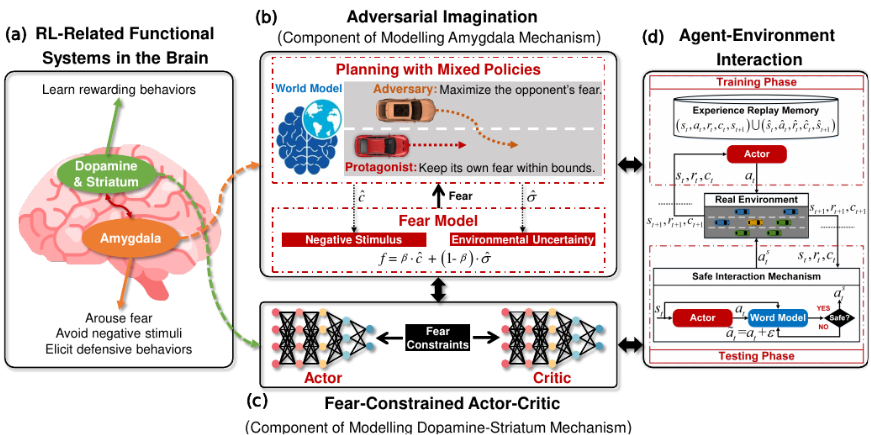
To put this system through its paces, the researchers tested it in a series of high-fidelity driving simulations featuring challenging, safety-critical scenarios:
- Sudden cut-ins and swerves by aggressive drivers
- Erratic pedestrians jaywalking into traffic
- Sharp turns and blind corners with limited visibility
- Slick roads and poor weather conditions
Across these tests, the FNI-RL-equipped vehicles demonstrated remarkable safety performance, consistently outperforming human drivers and traditional reinforcement learning (RL) techniques to avoid collisions and practice defensive driving skills.
In one striking example, the FNI-RL system successfully navigated a sudden, high-speed traffic merger with a 90% success rate, compared to just 60% for a state-of-the-art RL baseline.
It even achieved safety gains without sacrificing driving performance or passenger comfort.
In other tests, the researchers probed the FNI-RL system’s ability to learn and generalize defensive strategies across driving environments.
In a simulation of a busy city intersection, the AI learned in just a few trials to recognize the telltale signs of a reckless driver – sudden lane changes, aggressive acceleration – and pre-emptively adjust its own behavior to give a wider berth.
Remarkably, the system was then able to transfer this learned wariness to a novel highway driving scenario, automatically registering dangerous cut-in maneuvers and responding with evasive action.
This demonstrates the potential of neurally-inspired emotional intelligence to enhance the safety and robustness of autonomous driving systems.
By endowing vehicles with a “digital amygdala” tuned to the visceral cues of road risk, we may be able to create self-driving cars that can navigate the challenges of the open road with a fluid, proactive defensive awareness.
Towards a science of emotionally-aware robotics
While recent AI advancements have relied on brute-force computational power, researchers are now drawing inspiration from human emotional responses to create smarter and more adaptive artificial systems.
This paradigm, named “bio-inspired AI,” extends beyond self-driving cars to fields like manufacturing, healthcare, and space exploration.
There are many exciting angles to explore. For example, robotic hands are being developed with “digital nociceptors” that mimic pain receptors, enabling swift reactions to potential damage.
In terms of hardware, IBM’s bio-inspired analog chips use “memristors” to store varying numerical values, reducing data transmission between memory and processor.
Similarly, researchers at the Indian Institute of Technology, Bombay, have designed a chip for Spiking Neural Networks (SNNs), which closely mimic biological neuron function.
Professor Udayan Ganguly reports this chip achieves “5,000 times lower energy per spike at a similar area and 10 times lower standby power” compared to conventional designs.
These advancements in neuromorphic computing bring us closer to what Ganguly describes as “an extremely low-power neurosynaptic core and real-time on-chip learning mechanism,” key elements for autonomous, biologically inspired neural networks.
Combining nature-inspired AI technology with architectures informed by natural emotional states like fear or curiosity could thrust AI into an entirely new state of being.
As researchers push those boundaries, they’re not just creating more efficient machines – they’re potentially birthing a new form of intelligence.
As this line of research evolves, autonomous machines might roam the world among us, reacting to unpredictable environmental cues with curiosity, fear, and other emotions considered distinctly human.
The impacts? That’s another story altogether!
The post Is “fear” the key to building more adaptable, resilient, and natural AI systems? appeared first on DailyAI.