AI models face collapse when trained on AI-generated data, study finds
A new study published in Nature reveals that AI models, including large language models (LLMs), rapidly degrade in quality when trained on data generated by previous AI models. This phenomenon, termed “model collapse,” could erode the quality of future AI models, particularly as more AI-generated content is released onto the internet and, therefore, recycled and reused in model training data. Investigating this phenomenon, researchers from the University of Cambridge, University of Oxford, and other institutions conducted experiments showing that when AI models are repeatedly trained on data produced by earlier versions of themselves, they begin to generate increasingly nonsensical outputs. The post AI models face collapse when trained on AI-generated data, study finds appeared first on DailyAI.
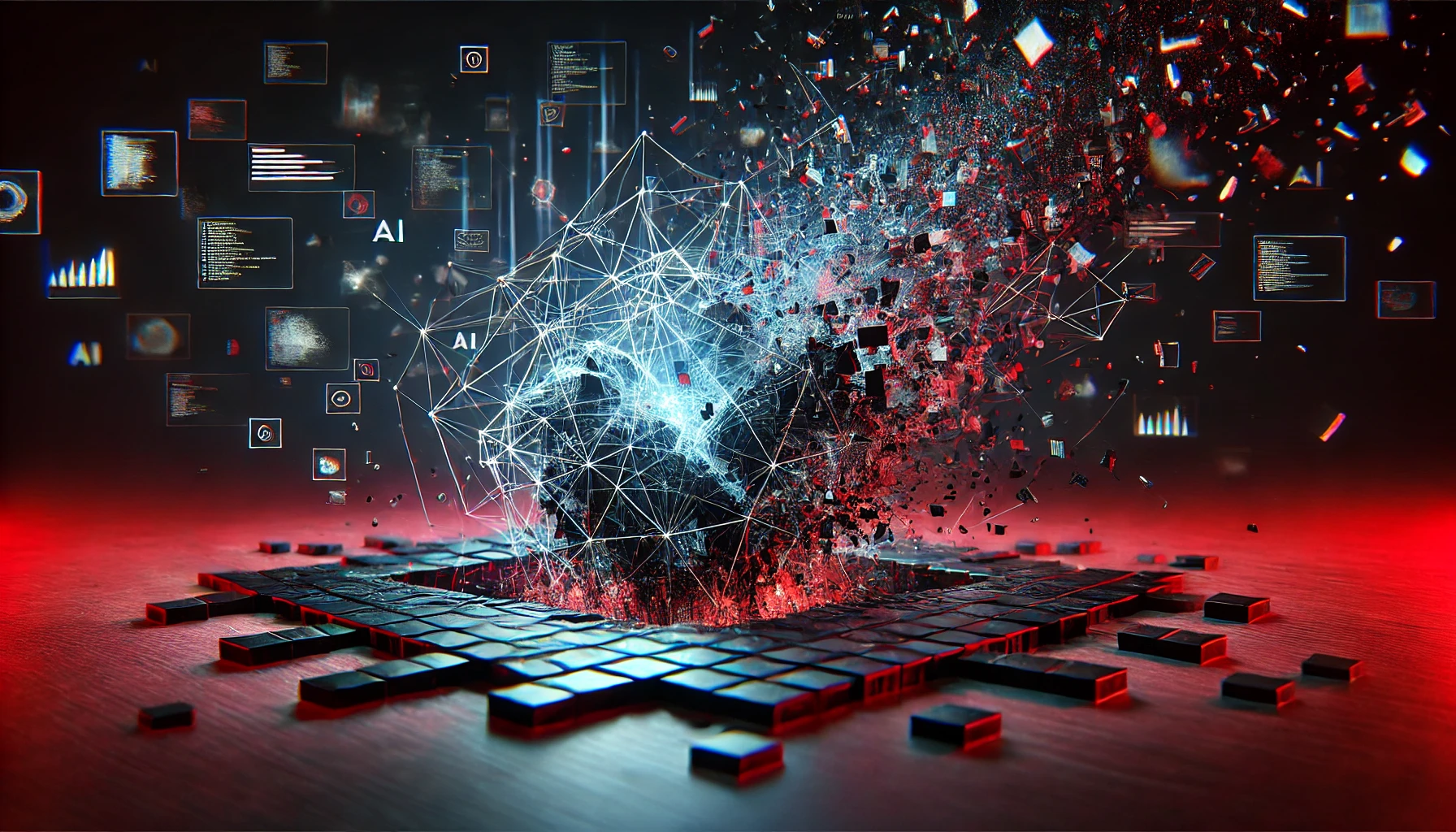
A new study published in Nature reveals that AI models, including large language models (LLMs), rapidly degrade in quality when trained on data generated by previous AI models.
This phenomenon, termed “model collapse,” could erode the quality of future AI models, particularly as more AI-generated content is released onto the internet and, therefore, recycled and reused in model training data.
Investigating this phenomenon, researchers from the University of Cambridge, University of Oxford, and other institutions conducted experiments showing that when AI models are repeatedly trained on data produced by earlier versions of themselves, they begin to generate increasingly nonsensical outputs.
This effect was observed across different types of AI models, including language models, variational autoencoders, and Gaussian mixture models.
To demonstrate the impacts of model collapse, the research team conducted a series of experiments using different AI architectures.
In one key experiment with language models, they fine-tuned the OPT-125m model on the WikiText-2 dataset and then used it to generate new text. This AI-generated text was then used to train the next “generation” of the model, and the process was repeated.
Results showed that the models began producing increasingly improbable and nonsensical text over successive generations.
By the ninth generation, the model was generating complete gibberish, such as listing multiple non-existent types of “jackrabbits” when prompted about English church towers.
Three main sources of error were identified:
- Statistical approximation error: Arises due to the finite number of samples used in training.
- Functional expressivity error: Occurs because of limitations in the model’s ability to represent complex functions.
- Functional approximation error: Results from imperfections in the learning process itself.
The researchers also observed that the models began to lose information about less frequent events in their training data even before complete collapse.
This is alarming, as rare events often relate to marginalized groups or outliers. Without them, models risk concentrating their responses across a narrow spectrum of ideas and beliefs, reinforcing biases.
Compounding this effect, a study by Dr. Richard Fletcher, Director of Research at the Reuters Institute for the Study of Journalism, recently found that nearly half (48%) of the most popular news sites worldwide are now inaccessible to OpenAI’s crawlers, with Google’s AI crawlers being blocked by 24% of sites.
Therefore, AI models have access to a smaller pool of high-quality, recent data than they once did, potentially increasing the risk of training on sub-standard or outdated data.
AI companies are aware of this, hence why they’re striking deals with news companies and publishers to secure a steady stream of high-quality, human-written, topically relevant information.
“The message is, we have to be very careful about what ends up in our training data,” study co-author Zakhar Shumaylov from the University of Cambridge told Nature. “Otherwise, things will always, provably, go wrong.”
Solutions to model collapse
Regarding solutions, researchers conclude that maintaining access to original, human-generated data sources will be vital for the long-term viability of AI systems.
They also suggest that tracking and managing AI-generated content will be necessary to prevent it from contaminating training datasets.
Potential solutions proposed by the researchers include:
- Watermarking AI-generated content to distinguish it from human-created data
- Creating incentives for humans to continue producing high-quality content
- Developing more sophisticated filtering and curation methods for training data
- Exploring ways to preserve and prioritize access to original, non-AI-generated information
Model collapse is a real problem
This study is far from the only one exploring model collapse.
Not long ago, Stanford researchers compared two scenarios in which model collapse might occur: one in which each new iteration’s training data fully replaced the previous data and another in which new synthetic data was added to the existing dataset.
The results showed that when data was replaced, model performance deteriorated rapidly across all tested architectures.
However, when data was allowed to “accumulate,” model collapse was largely avoided. The AI systems maintained their performance and, in some cases, showed improvements.
So, instead of discarding the original real data and using only synthetic data to train the model, the researchers combined both.
The next iteration of the AI model is trained on this expanded dataset, which includes both the original real data and the newly generated synthetic data, and so on.
Thus, model collapse isn’t a foregone conclusion – it depends on how much AI-generated data is in the set and the ratio of synthetic to authentic data.
If and when model collapse starts to become evident in frontier models, you can be certain that AI companies will be scrambling for a long-term solution.
The post AI models face collapse when trained on AI-generated data, study finds appeared first on DailyAI.